Research Project:
A Corpus for Persuasion Techniques in Multimodal Data
The Tanbih team received funding of 85K $ from Meta. The goal is to create a process for flagging misinformation and propaganda on social media platforms
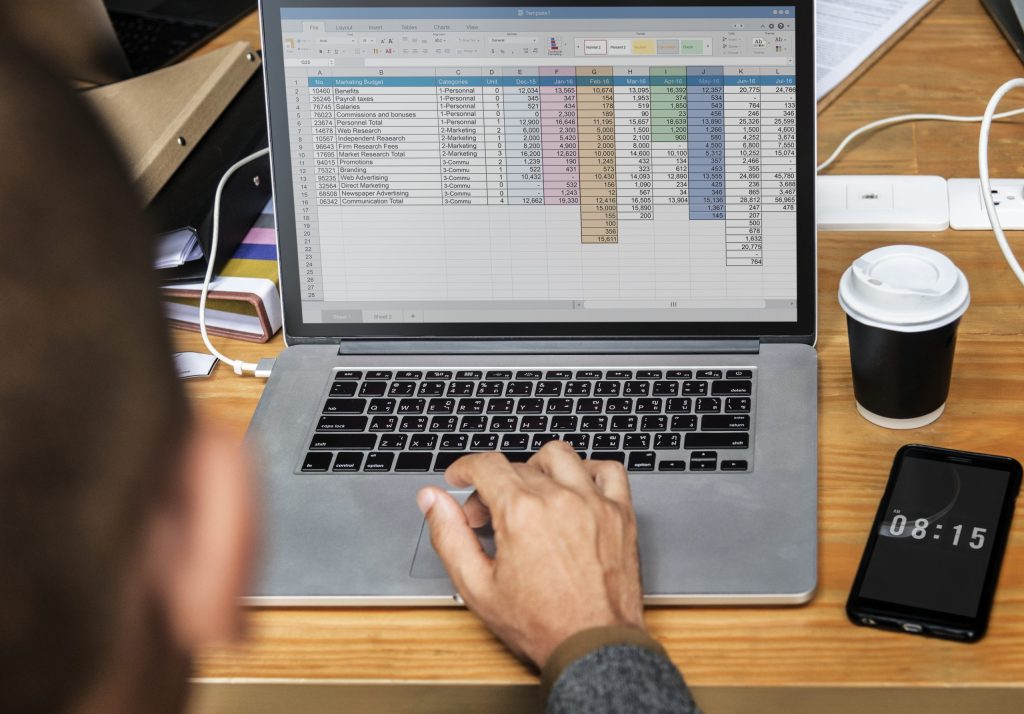
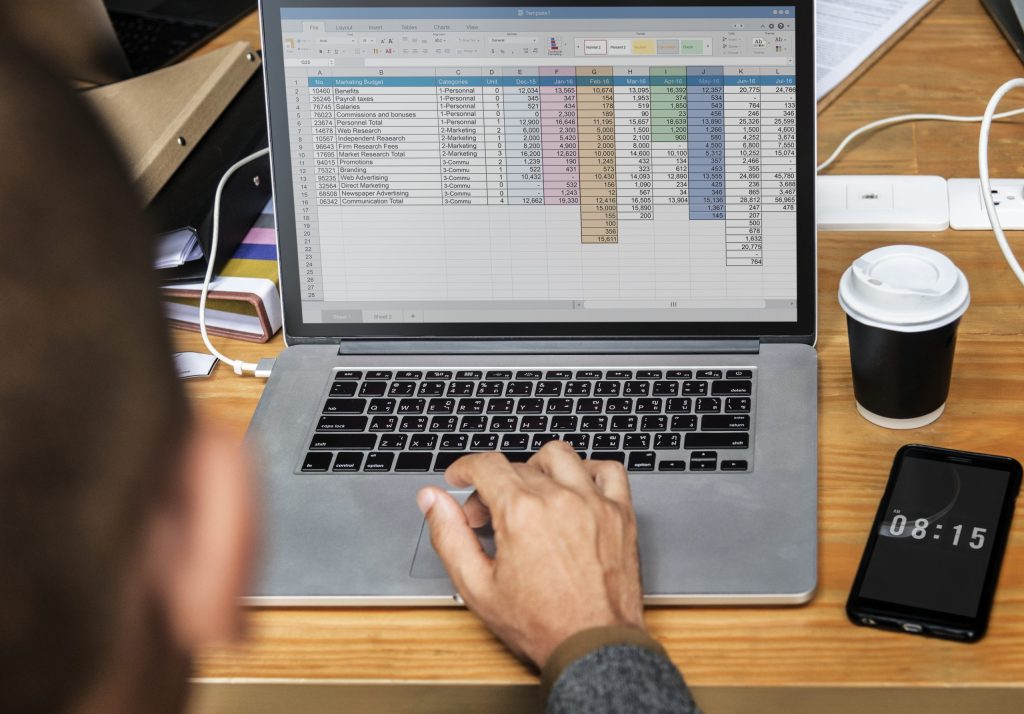
Research Purpose
- Identifying the techniques used in inflammatory, misinformed, and propagandist, multimodal social media posts.
Creating a set of annotation protocols and guidelines for annotating the texts and images in inflammatory and propagandist multimodal social media posts.
Creating a machine learning algorithm that can detect inflammatory and propagandist multimodal social media posts. All Foreground IP will be open sourced for public access per the SRA.
Research Description
Visual cues have been shown to be an important manipulator in fake news propaganda and are regularly used as persuasive vehicles for misinformation [Shu et al., 2017]. During the 2016 election campaign, malicious accounts (social bots, cyborg, trolls) on Facebook and Twitter used sensational images to provoke anger or other emotional responses from consumers [Guo et al., 2019]. Moreover, studying the “meme archive” on FactCheck.org indicates that Internet memes (“Memes”), as multimodal entities, represent a major part of misinformation campaigns.
The goal of this Research is to encourage the research community to study the problem of misinformation by providing models and techniques to detect deception in social media content by (i) creating a corpus of various manipulative, misleading and propagandist images and text, (ii) constructing a machine learning algorithm to analyze such images and text, and (iii) open source publishing the Foreground IP for public access.
A secondary goal of the research performed under this SOW is to allow Sponsor to proactively demote contents with high deception scores and nominate them for fact-checking/hate speech detection before they become viral. HBKU/QCRI believes that the deceptive techniques could help explain the demotion process, e.g., by highlighting parts of the text or of the image that use certain types of deceptive techniques such as “Appeal to Fear”, “Loaded Language”, etc. This can make it clear to human fact-checkers why the image has been suggested for fact- checking/hate speech detection, or could be used to offer a similar explanation to the end user.
Resources Developed
~9,000 memes annotated.